Data Analytics Categories: Descriptive, Predictive, Prescriptive
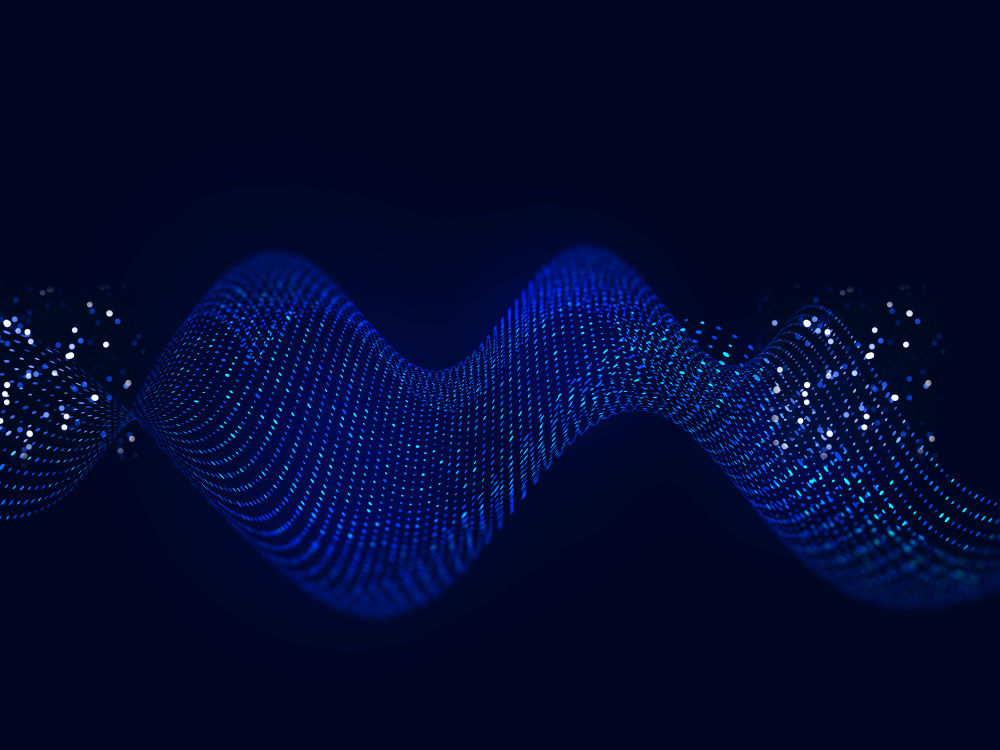
Data analytics is essential for informed decision-making in modern enterprises, particularly in sectors that handle large volumes of unstructured data, such as healthcare, finance, and government. This article explores three key categories of data analytics: descriptive, predictive, and prescriptive analytics, emphasizing their methodologies, applications, and the critical role they play in regulated environments.
Descriptive Analytics: Understanding What Happened
Descriptive analytics focuses on analyzing historical data to identify trends and patterns. It forms the foundation for understanding past events and behaviors, providing insights that enable better comprehension of operations.
Data is aggregated and summarized using visualization techniques and reporting tools to transform raw information into actionable insights. For instance, a dataset may be summarized to highlight key patterns, trends, or anomalies. Techniques such as calculating statistical measures (e.g., mean, median, standard deviation) offer a comprehensive understanding of data distribution, allowing analysts to identify outliers and variability.
Descriptive analytics is crucial for surfacing operational insights, ensuring data quality, and laying the groundwork for advanced analytics processes by enhancing the integrity and reliability of the data.
Predictive Analytics: Forecasting Future Trends
Predictive analytics utilizes historical data to forecast future outcomes by employing statistical models and machine learning techniques. It builds on the insights gained from descriptive analytics to anticipate future behaviors or events.
Common modeling techniques include regression analysis, time series forecasting, and machine learning algorithms like decision trees and neural networks. These methods detect patterns and relationships within the data to make informed predictions about future occurrences. Techniques such as linear regression are effective for predicting continuous variables, while classification algorithms can be applied to categorize data.
Predictive analytics is instrumental in enabling proactive decision-making. For example, forecasting trends in demand or resource allocation can significantly improve operational efficiency and readiness, particularly in sectors that require anticipatory measures.
Prescriptive Analytics: Recommending Actions
Prescriptive analytics goes beyond predicting future events by recommending actions based on data-driven insights. It optimizes decision-making processes by suggesting the most effective course of action under specific circumstances.
Optimization models and complex algorithms are employed to determine the best solutions to a given problem. For instance, linear programming may be used to allocate resources optimally. Simulation techniques such as Monte Carlo simulations are employed to assess the impact of different decisions under uncertain conditions, making it possible to evaluate multiple scenarios.
Prescriptive analytics is essential for addressing complex decision-making challenges, offering actionable recommendations that help enterprises achieve optimal outcomes. Its applications range from resource management to regulatory compliance strategies, ensuring both efficiency and adherence to industry standards.
Technical Considerations for Effective Implementation
The successful implementation of descriptive, predictive, and prescriptive analytics depends on several technical factors that directly affect the quality and scalability of the insights generated:
- Data Quality: Maintaining high-quality data is paramount. Proper data preprocessing, including cleaning, normalization, and validation, ensures the data is accurate, consistent, and complete. Reliable data is essential for generating meaningful analytics results, particularly when dealing with unstructured data sources.
- Scalable Infrastructure: Handling vast amounts of unstructured data requires a robust and scalable infrastructure. Cloud-based platforms offer the flexibility and computational power necessary for processing and analyzing large datasets efficiently, ensuring that analytics workflows can be scaled according to the enterprise's needs.
- Advanced Tooling: Leveraging automated tools for data labeling and categorization facilitates precise organization and filtering of unstructured data. This step is critical for enabling advanced analytics processes, particularly in environments where large datasets are the norm.
- Integration of Domain Expertise: Incorporating domain knowledge into the analytics process enhances the relevance and applicability of the insights generated. This is especially important in regulated industries, where compliance, accuracy, and contextual understanding are critical to ensuring that analytics outputs are not only correct but also actionable.
Case Study: Application in Healthcare Analytics
To illustrate the practical implementation of these analytics categories, consider a healthcare institution seeking to reduce hospital readmissions.
Using descriptive analytics, the institution first analyzed five years of historical data on patient readmissions. Patterns emerged, showing high readmission rates for specific demographics, particularly among elderly patients with chronic conditions such as diabetes and heart disease. This insight provided a solid foundation for deeper analysis.
Next, a predictive analytics model using logistic regression was developed. This model incorporated patient demographics, medical history, and treatment data to predict the likelihood of readmission. The model achieved an accuracy rate of 85%, allowing the hospital to identify high-risk patients who were most likely to be readmitted within 30 days of discharge.
Building on this, prescriptive analytics was applied to recommend personalized care plans for high-risk patients. The institution used an optimization algorithm to suggest follow-up schedules, medication adjustments, and targeted interventions, such as remote monitoring for patients with poor medication adherence or at-home care services for patients lacking family support. Over the course of six months, this approach resulted in a 20% reduction in readmission rates, while improving patient satisfaction and operational efficiency.
Strategic Importance of Data Analytics
Descriptive, predictive, and prescriptive analytics together form a robust data strategy that allows enterprises to derive actionable insights from large volumes of data. Descriptive analytics provides a thorough understanding of historical data, which in turn primes the data for future predictions through predictive analytics. Finally, prescriptive analytics delivers recommendations that help organizations optimize their decision-making processes.
In regulated industries such as healthcare, financial services, and government, the integration of these analytics techniques is vital for ensuring compliance, improving operational efficiency, and guiding strategic decisions. The ability to transform raw data into strategic insights ensures that enterprises remain agile and competitive in a data-driven world.
Empowering Enterprises Through Analytics
Mastering the application of descriptive, predictive, and prescriptive analytics enables organizations to turn data into a strategic asset. By investing in scalable infrastructure, maintaining data quality, and incorporating domain expertise, enterprises can leverage these analytics techniques to drive innovation, optimize operations, and remain compliant in complex regulatory environments. The ongoing evolution of data analytics presents significant opportunities for enterprises to enhance their capabilities, ensuring sustained growth and success in their respective fields.