Data Management Tool: Optimize Your Data Handling with the Latest Tech
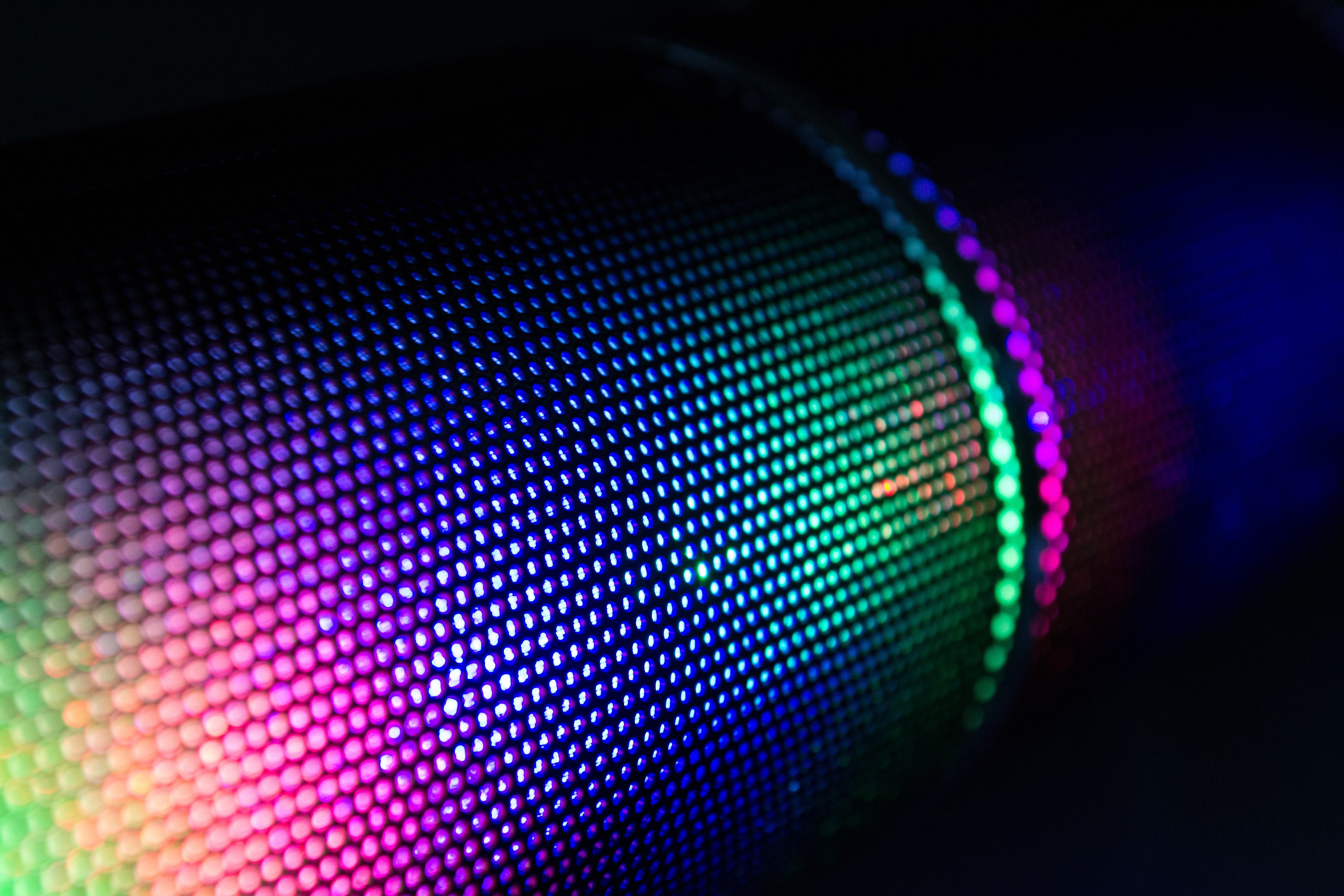
Understanding the Importance of Data Management in Enterprises
In today's digitized economy, data reigns supreme. For large organizations operating in sectors such as finance, healthcare, and government, effective data management is more than a mere advantage – it is fundamental to their success. Data represents invaluable insights into consumers, trends, and operational efficiencies. However, to unlock these insights, data needs to be appropriately gathered, stored, processed, and analyzed. This is where the importance of data management emerges.
Data-driven decision making allows businesses to cater to their consumer's needs more effectively. Strategies built upon a solid foundation of diverse, accurate, and timely data can predict market movements, anticipate consumer behavior, and deliver competitive products and services.
Additionally, organizations amassing large volumes of unstructured data—which includes emails, social media posts, customer feedback, etc.—necessitates data management systems up to the task. Without structured analysis and interpretation, unstructured data is as good as indecipherable, leaving its potential untapped.
Data management also ties to regulatory compliance. Laws such as the General Data Protection Regulation (GDPR) and the California Consumer Privacy Act (CCPA) require that businesses handle data ethically and transparently. Failure to comply can result in hefty fines. More than that, data breaches can cause irreparable damage to a company's reputation. Therefore, strong data management strategies and tools are essential for legal compliance and maintaining trust in a data-conscious world.
Challenges of Traditional Data Management Approaches
Companies that have not yet modernized their data management protocols often collide head-on with operational inefficiencies. Traditional data systems, usually characterized by siloed databases and manual data entry, are not equipped to handle the rapidly growing volumes of digitally harvested data.
Inaccuracy is another significant challenge with outmoded data handling techniques. Manual data input is susceptible to human error, potentially corrupting critical data. Moreover, the lack of real-time updates may cause outdated information to persist in databases. This outdated or erroneous data can lead to poor strategic decisions, causing a ripple of negative effects throughout the organization.
Lastly, traditional data management is laborious and resource-intensive. IT staff may need to devote a significant portion of their time to mundane data entry or resolving data inconsistencies. This not only increases operational costs but also prevents them from taking on more value-adding tasks like enhancing security measures or optimizing software performance.
Navigating these challenges requires a shift in approach. And with the advent of perpetually evolving technology, organizations may find the right support in AI-powered solutions. Enter, Data Management Tools.
Evolution of Data Management: The Rise of AI and Machine Learning
A new era in data management has dawned, thanks to the continuous advancements in artificial intelligence (AI) and machine learning (ML). By implementing AI and ML into data management tools, the process of storing, processing, and analyzing data is exponentially improved.
AI-driven data management tools constantly learn from the patterns and datasets they interact with, increasing their efficiency over time. They can automate numerous tasks that were previously handled manually, eradicating human error and significantly reducing operational costs. On top of that, they can effortlessly organize, process, and analyze enormous data volumes, garnering intelligent insights rapidly.
In an ML-infused data management environment, predicting trends, understanding customer behavior, and spotting anomalies becomes seamless. Data predictions and real-time decision-making allot businesses a competitive edge in the ever-changing market. Machine learning algorithms can parse through vast, complex datasets to extract specific data points, uncover hidden correlations, and predict future outcomes.
Perhaps one of the most crucial benefits of integrating AI and ML into data management is the system's ability to self-regulate and optimize. These tools can self-identify issues such as data quality and data redundancy and make real-time modifications. They're not simply reactive; they can preemptively address data discrepancies before they snowball into substantial problems.
The Anatomy of a Modern Data Management Tool
When considering an upgrade to a modern data management tool, understanding its defining features is imperative.
Firstly, a potent data management tool should be adept at data organization. It should ensure the data in the system is correctly structured and easily accessible. From de-duplicating data to streamlining the metadata, these features ensure data retrieval is precise and efficient.
While we're discussing precision, data accuracy is a crucial feature. Data is only as valuable as it is accurate. With AI and ML algorithms, modern data management tools can continuously clean and verify data, maintaining the database's integrity.
Data recovery is another fundamental aspect. The tool should have robust backup and recovery systems to handle any data loss incidents. Given the valuable nature of enterprise data, a missing dataset can have significant financial and operational implications.
Data security is, of course, a critical consideration. With data breaches making headlines, and laws enforcing stringent data privacy guidelines, a data management tool should be solid on defending against unauthorized accesses and data leaks.
Moreover, regulatory compliance functionalities can be a life-saver for heavily regulated industries. Tools offering automated report generation, audit trails, and data governance can significantly ease compliance management.
Vendor support and tool scalability need to be considered. As businesses grow and evolve, their data management needs would change – sometimes abruptly. The chosen data management tool should be equipped to adapt to the changing needs swiftly and efficiently.
A peek into the market reveals significant players such as IBM's DataOps, Oracle's Data Management Platform, and Microsoft Azure's Purview. Each offers a suite of data management services that cater to diverse enterprise needs.
Impact of Cloud-Based Data Management
Emerging distinctly amidst numerous advancements in data management is the transition towards cloud-based solutions. Cloud computing has dramatically altered data management landscapes, offering a myriad of benefits over traditional, on-premise systems.
Cloud-based data management tools deliver unmatched scalability and flexibility, capable of quickly adapting to changing business needs. Data storage can be expanded or reduced at will, addressing the rigid constraints linked with physical servers and storage facilities.
Moreover, accessibility is a key strength of cloud-based data tools. Authorized personnel can access necessary data from anywhere, anytime, expediting decision-making processes that rely on real-time data insights.
Then there's the question of data security in the cloud. Despite initial concerns, the reality is that top cloud service providers now offer robust security protocols, including encryption, identity management, and access controls. This level of security is often higher than most companies can achieve in-house.
Lastly, cloud providers often ensure their platforms are compliant with common regulations. For organizations in highly regulated industries, this shared responsibility can alleviate the pressure of ensuring comprehensive compliance.
The Future of Data Management: Automation, Predictive Analytics, and More
What’s next for data management? The future promises further exploration of automation and predictive analytics.
Progress in AI and ML algorithms will allow for enhanced automation of data management tasks. Not only does this speed up operations, but it also creates a more efficient system capable of handling high volumes of data with minimal human intervention. Imagine a system equipped to self-detect errors and inconsistencies, and make modifications, all in real-time.
Predictive analytics, an already burgeoning field, stands to become even more integral in future data management strategies. With the capacity to predict trends and proactively safeguard against potential business risks, predictive analytics will transform the way enterprises interact with and use their data.
Emerging technologies like edge computing and the Internet of Things (IoT) will also significantly influence data management. The IoT has already begun to revolutionize data collection, creating data streams from a diverse range of sources in real-time. Data generated from the IoT will require innovative storage, processing, and analysis techniques, introducing a new frontier in data management.
As these technologies evolve and others emerge, the demand for robust, sophisticated data management tools will also increase. Consequently, the focus of development in the sector will likely be on creating versatile, scalable tools equipped to handle dynamic, complex operations efficiently.
Indeed, the future looks brighter and increasingly data-driven. As enterprises navigate their path, their success will hinge on their adoption, optimization, utilization of the most fitting data management tools. Often, the future is unpredictable, but in this domain, one forecast appears certain: the continued growth and increasing sophistication of data management tools.
How Enterprises Can Choose the Best Data Management Tool for Their Needs
Selecting a data management tool is a significant undertaking that directly influences an enterprise's operational efficiency and strategic success. Hence, the choice requires careful evaluation of numerous aspects.
To start with, enterprises need to assess their specific data requirements. The nature and volume of data, the desired analytics, and the unique industry-specific demands – all these should determine the functionality a tool needs to offer. One-size-fits-all doesn’t apply here; a financial institution’s requirements will vary greatly from a healthcare organization.
A second important aspect is the tool's scalability. As businesses grow or shift directions, the demand on their data management system will shift as well. The chosen tool should be capable of accommodating these changes to avoid any potential operational hiccups.
Usability also plays a crucial role. A tool that is difficult to master or requires extensive IT involvement to be functional will only bottleneck operations. Enterprises need to opt for tools with intuitive interfaces and, preferably, good vendor support.
As we've emphasized throughout, data security cannot be overlooked. The tool has to offer robust security measures that can be scaled as per the risk assessment of the data being handled.
Similarly, regulatory compliance is a must. Enterprises operating under stringent regulatory laws need tools capable of generating necessary logs, reports, and audit trails for compliance.
Finally, cost comes into the picture. While investing in a data management tool is necessary, deciding how much to spend depends on available resources and projected return on investment. organizations need to analyze if the tool can truly deliver cost savings in the longer run.
Through meticulous evaluation and a deep understanding of their unique needs, enterprises can identify and implement a data management tool that streamlines their operations, safeguards their data, and enables informed, data-driven decision-making.
If you're interested in exploring how Deasie's data governance platform can help your team improve Data Governance, click here to learn more and request a demo.