Deploying Auto-Suggested Metadata
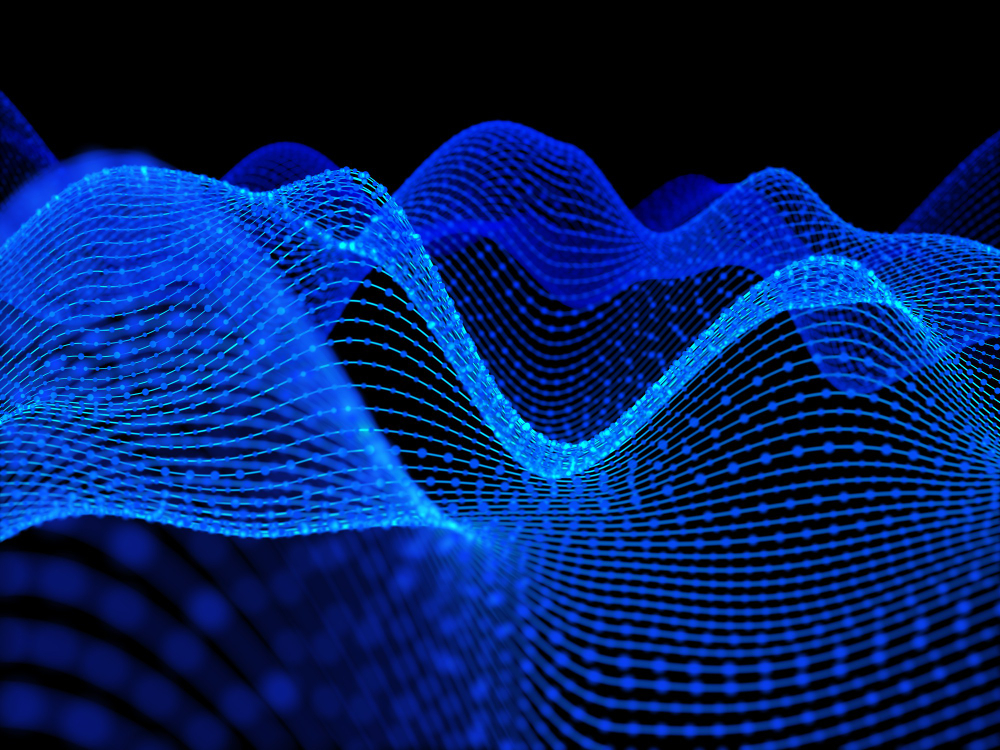
Auto-suggested metadata is revolutionizing how enterprises manage and utilize unstructured data. As organizations grapple with vast amounts of information, metadata serves as the foundation for efficient data cataloging, retrieval, and analysis. Deploying auto-suggested metadata systems can significantly enhance data management processes, particularly in regulated industries dealing with large data volumes. This article explores the technical dimensions, advantages, and practical applications of auto-suggested metadata systems.
Technical Foundations of Auto-Suggested Metadata
Metadata, often described as "data about data," conveys vital information about data objects, such as their origin, structure, and content. In the context of unstructured data, which lacks inherent organization, metadata is essential for imposing structured frameworks that facilitate data management. Generating metadata manually is laborious and prone to human error, particularly when dealing with expansive datasets.
Auto-suggested metadata leverages machine learning (ML), natural language processing (NLP), and AI algorithms to automate the generation and suggestion of relevant metadata tags. These systems can:
- Analyze Content: ML and NLP algorithms parse through text, images, videos, and other unstructured data types to extract meaningful insights. Techniques like named entity recognition (NER), image classification, and sentiment analysis are employed to identify pertinent attributes of the data.
- Contextual Understanding: These algorithms comprehend the context by examining surrounding text, historical usage patterns, and domain-specific knowledge. This context-awareness allows for accurate and context-sensitive metadata suggestions.
- Iterative Learning: Auto-suggested metadata systems continuously learn from user interactions and feedback. As users accept, modify, or reject suggested tags, the system refines its algorithms to enhance future predictions.
Advantages of Auto-Suggested Metadata
The deployment of auto-suggested metadata offers numerous technical and operational advantages:
- Enhanced Data Discoverability: Accurate metadata improves data discoverability by enabling efficient searching and indexing. Users can locate relevant data quickly, significantly reducing the time spent on data retrieval activities.
- Operational Efficiency: Automating metadata generation streamlines data management workflows. It reduces the reliance on manual tagging, freeing up human resources for higher-value tasks.
- Consistency and Accuracy: Human annotators are susceptible to inconsistencies and errors, especially when handling large datasets. Auto-suggested metadata ensures consistent and precise tagging, thereby standardizing data across the organization.
- Scalability: These systems are designed to handle large volumes of data, making them ideal for enterprises dealing with massive datasets. Auto-suggested metadata tools scale effortlessly, accommodating growing data needs without compromising performance.
Deep Dive: Case Study on Auto-Suggested Metadata in Financial Services
To illustrate the practical application and impact of auto-suggested metadata, consider a case study in the financial services sector. Financial institutions accumulate colossal amounts of unstructured data from various sources, including transaction records and regulatory documents.
1. Implementation Approach:
- Data Integration: The organization's unstructured data, stored across multiple cloud environments, is integrated into a centralized repository. A robust data ingestion framework ensures seamless data flow into the auto-suggested metadata system.
- Algorithm Selection: Advanced NLP algorithms, tailored for financial terminology and regulatory compliance, are deployed. These algorithms are trained on a diverse dataset encompassing historical records, industry-specific documentation, and real-time data feeds.
- User Feedback Loops: An interactive interface allows finance professionals to review and provide feedback on suggested metadata tags. This feedback loop is crucial for refining the accuracy and applicability of the metadata suggestions.
2. Results and Analysis:
- Improved Data Access: The deployment resulted in a significant reduction in the time required to retrieve relevant documents for compliance reporting. Auto-suggested metadata facilitated swift access to vital information, enhancing operational efficiency and decision-making.
- Regulatory Compliance: Accurate and consistent metadata ensured that all documents met stringent regulatory requirements. The system's ability to suggest contextually relevant regulatory tags reduced the risk of non-compliance significantly.
- Cost Savings: The automation of metadata generation led to substantial cost savings by reducing labor costs associated with manual tagging, translating to significant resource optimization.
Technical Considerations and Best Practices
Deploying auto-suggested metadata requires careful planning and execution. Key technical considerations and best practices include:
- Algorithm Training: Effective auto-suggested metadata systems rely on well-trained algorithms. This necessitates the use of high-quality training datasets reflective of the enterprise’s specific domain and data characteristics.
- Customization: Off-the-shelf solutions may not always meet the unique needs of an organization. Customizing algorithms to align with specific industry terminology, compliance standards, and operational workflows enhances the relevance and effectiveness of metadata suggestions.
- User Integration: Incorporating user feedback into the system is essential. An interactive interface that allows users to review and adjust suggested tags not only improves accuracy but also fosters user trust and adoption.
- Scalability and Performance: Ensure that the system is scalable and can handle the growing volume and complexity of data. Performance metrics, such as latency and throughput, should be monitored and optimized continuously.
Emphasizing the Strategic Role of Auto-Suggested Metadata Systems
In our opinion, deploying auto-suggested metadata systems is a strategic imperative for modern enterprises. These systems not only enhance data management efficiency but also drive substantial improvements in data quality, accessibility, and compliance. As data volumes continue to surge, the relevance of auto-suggested metadata will grow, cementing its role as a cornerstone of effective data management strategies.
By integrating auto-suggested metadata into their data management practices, organizations can unlock the full potential of their unstructured data, transforming it into a valuable asset that drives innovation, operational efficiency, and competitive advantage.