Empowering Users with No-Code Data Labeling
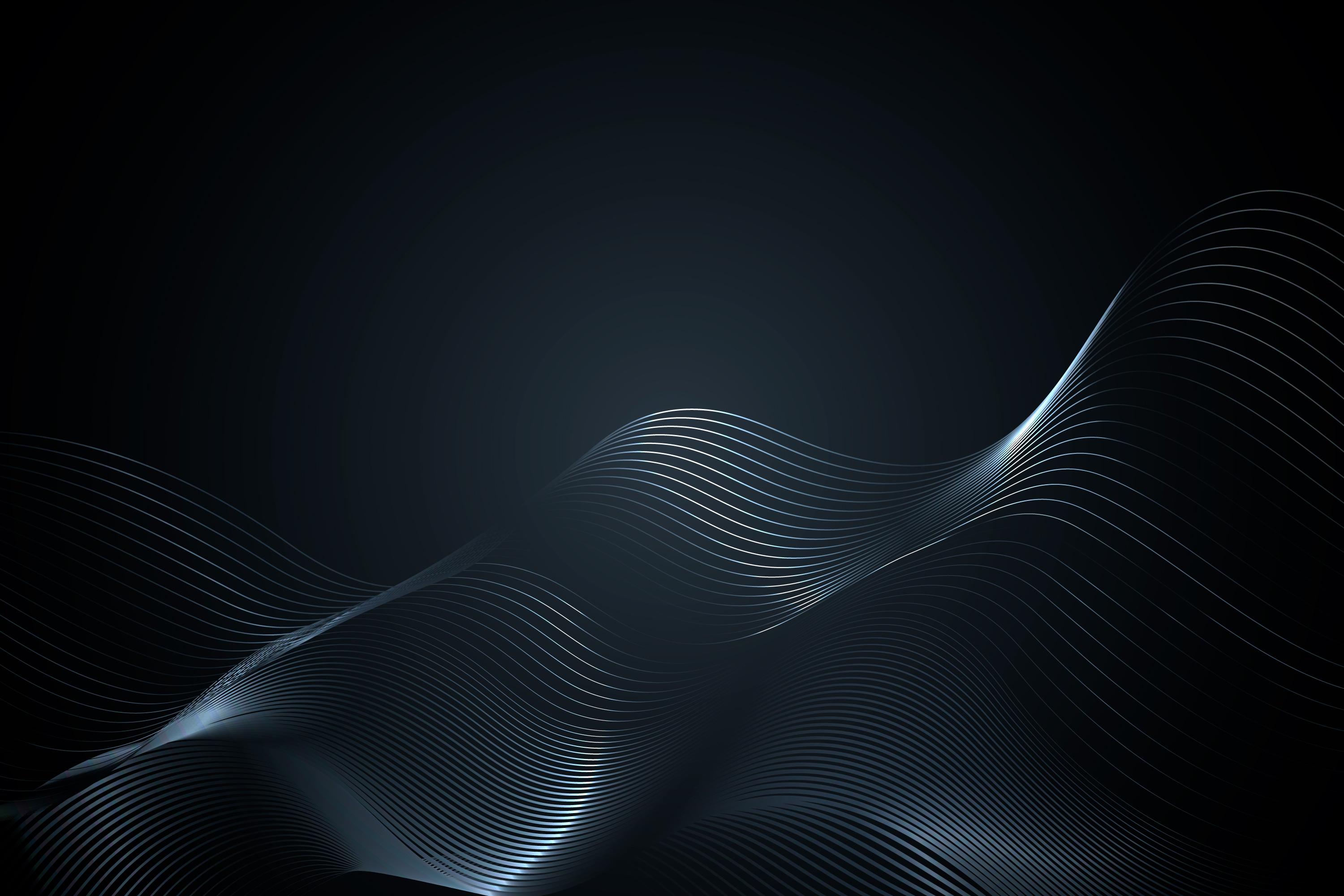
The complexity and cost associated with traditional data labeling methods often hinder enterprises from fully harnessing the potential of machine learning and artificial intelligence, particularly when dealing with large volumes of unstructured data. No-code data labeling solutions have emerged as a transformative approach, democratizing the data annotation process and enabling a wider range of users to participate without the need for specialized technical skills. This article explores the technical foundations, practical impacts, and strategic implications of adopting no-code data labeling solutions.
Technical Foundations of No-Code Data Labeling
No-code data labeling platforms offer several functionalities that streamline the labeling process:
- Visual Interface: Users interact with data through drag-and-drop features, dropdown menus, and visual tools. These platforms often include templates for different data types, enabling easier annotation without deep technical knowledge.
- Automated Label Suggestions: Machine learning algorithms provide label suggestions based on existing annotated data, thereby accelerating the annotation process and improving over time as they are exposed to more examples.
- Collaborative Tools: Features like role-based access control, audit logs, and versioning help maintain data integrity and facilitate collaboration among multiple users.
Practical Impact on Annotation Workflow
In our opinion, no-code data labeling can significantly enhance the efficiency and effectiveness of the annotation process. The advantages include:
- Reduction in Dependency on Technical Teams: By enabling non-technical users to participate in data labeling, organizations can allocate their technical teams to more complex tasks, such as algorithm development and data analysis.
- Error Minimization: Visual interfaces and automated validation checks reduce the likelihood of human errors, thereby improving the quality of training data.
Enhanced Case Study: No-Code Data Labeling in Healthcare
Consider a healthcare organization aiming to build a predictive model for early diagnosis from retinal images. Traditionally, this process would require specialized medical professionals to label thousands of images, which is both time-consuming and costly.
Workflow Implementation
- Platform Selection and Setup: The organization selected a no-code data labeling platform that supported image annotation with an easy-to-use visual interface, including pre-annotated templates specifically designed for medical imaging. The platform's intuitive setup meant minimal onboarding time for medical professionals.
- Annotation Process: Medical professionals were able to annotate images by selecting predefined labels through a straightforward drag-and-drop interface. The platform's automated suggestion feature highlighted potential regions of interest, which annotators could then confirm, modify, or reject based on their expertise. This feature reduced the cognitive load and sped up the annotation process.
- Verification and Quality Control: To ensure high accuracy, the platform enabled multiple medical professionals to review and cross-verify annotations. Automated checks were in place to flag inconsistent annotations, requiring further review. This step ensured that the final dataset was of the highest quality.
- Compliance and Collaboration: Collaborative tools allowed multiple professionals to work simultaneously, with role-based access control ensuring that only authorized personnel could modify annotations. Real-time logging of changes facilitated audit trails, which are crucial for maintaining compliance with healthcare regulations. The platform's versioning system allowed easy rollback to previous states if necessary, providing an additional layer of data security.
Observations and Outcomes
In our view, the project achieved significant improvements. The total annotation time was reduced by 50%, and the accuracy of the labels saw notable enhancements. The availability of pre-annotated templates and automated suggestions enabled medical professionals to focus on validating and refining annotations rather than starting from scratch. The collaborative tools not only improved the efficiency of the workflow but also reinforced the accuracy and consistency of the dataset.
Challenges and Considerations
Despite its advantages, implementing no-code data labeling in an enterprise setting requires addressing several challenges:
- Scalability: Ensuring the platform can scale with growing data volumes is essential, involving integration with cloud storage solutions and optimization of data pipelines.
- Customization: Some annotation tasks may require custom features. Evaluating whether the platform allows for sufficient customization through extensions or APIs is crucial.
- Quality Control: Establishing stringent quality control mechanisms, such as periodic audits and cross-validation by multiple annotators, is critical to maintain high data quality.
Strategic Implications for Enterprises
In our opinion, no-code data labeling presents a strategic advantage for enterprises looking to accelerate their AI and machine learning initiatives. By lowering the barriers to entry, these platforms democratize access to data annotation, empowering a broader segment of the workforce to contribute to AI projects. The resulting time and cost savings, coupled with enhanced data quality, enable organizations to scale their AI operations more efficiently.
As data continues to grow in complexity and volume, embracing no-code data labeling will be pivotal in maintaining a competitive advantage. Integrating these platforms into data annotation workflows ensures that data handling practices evolve in tandem with the growing demands of modern AI systems. This positions enterprises to leverage the full potential of their data assets, driving innovation and operational efficiency.