Essential Practices for a Robust Metadata Framework
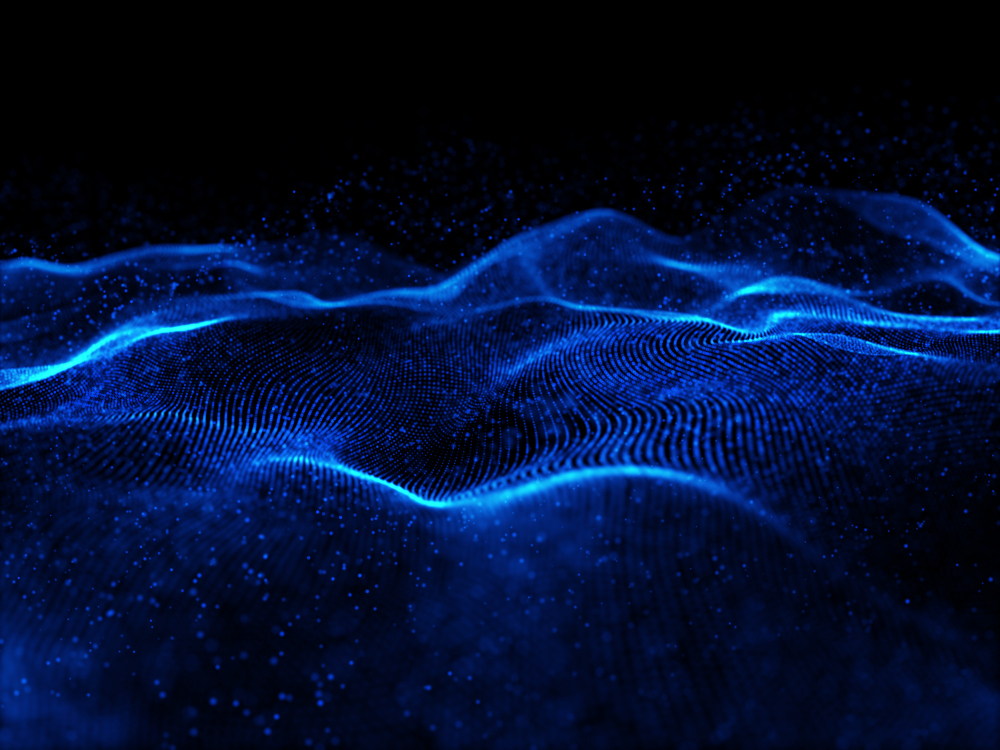
Metadata plays a pivotal role in managing and leveraging data, especially when dealing with unstructured information. Establishing a robust metadata framework involves certain practices to streamline data management, ensure data quality, and enhance the effectiveness of machine learning models. This article provides a technical examination of these practices, offering insights grounded in our experiences and real-world applications.
Understanding Metadata and Its Importance
Metadata is essentially data about data. It provides context, making datasets more understandable and usable. Effective metadata can clarify the origins, handling, and structure of data, facilitating better decision-making and more efficient data handling methodologies.
Key Components of a Robust Metadata Framework
- Standardization and Consistency
A standardized approach to metadata is essential. Standards such as Dublin Core, ISO 19115, or the Data Documentation Initiative (DDI) serve as guidelines, ensuring consistency across systems. Uniform metadata enhances interoperability and facilitates seamless data integration.
- Automated Metadata Generation and Tagging
Automation tools streamline the generation and tagging of metadata, significantly reducing manual effort and human error. Automated systems can extract relevant metadata attributes such as file type, creation date, author, and subject matter, thereby enriching datasets with minimal human intervention.
- Metadata Quality and Validation
Ensuring the quality of metadata is paramount, encompassing dimensions such as completeness, accuracy, consistency, and timeliness. Validation checks within the metadata framework can flag discrepancies and incomplete entries, maintaining the reliability and usability of metadata.
Dimensions for Measuring Metadata Quality
High-quality metadata enables precise data retrieval, efficient data integration, and improved machine learning model performance. Key dimensions include:
- Completeness: All necessary metadata elements are provided.
- Accuracy: Metadata entries accurately reflect the data content.
- Consistency: Uniformity in the representation of similar metadata across different datasets.
- Timeliness: Metadata is updated in sync with data changes.
Deep Dive: Metadata Framework Implementation in Financial Services
Case Study: Enhancing Data Governance in Financial Services
A financial institution encountered challenges in managing vast amounts of unstructured data, leading to inefficiencies and compliance risks. By adopting a robust metadata framework, the institution achieved notable improvements in data governance and operational efficiency, in our opinion.
- Framework Design: The institution employed a metadata framework incorporating relevant standards, ensuring a structured approach to managing financial data. Categories included transactional data, regulatory compliance, and customer information.
- Tool Integration: An automated system was used to annotate and tag unstructured data. This system enabled the extraction of critical metadata attributes, such as transaction timestamps and compliance flags, reducing manual intervention.
- Quality Assurance: A validation system continuously monitored metadata quality, flagging inconsistencies and missing elements, prompting corrective actions.
- Outcomes: The implementation led to a reduction in data retrieval times, improved compliance reporting, and enhanced data analytics capabilities, according to our observations.
Technical Considerations for Building a Metadata Framework
- Scalability: A robust metadata framework must be scalable to handle growing volumes of data. This involves selecting scalable storage solutions and indexing strategies that can accommodate increasing metadata entries without compromising performance.
- Interoperability: Utilizing standardized metadata formats and protocols facilitates seamless data exchange and integration, promoting a cohesive data ecosystem.
- Security and Privacy: Safeguarding metadata is as important as protecting the data itself. Implementing access controls, encryption, and audit trails within the framework ensures sensitive information remains secure and compliant with regulations.
The Strategic Imperative of Metadata Frameworks
In our opinion, investing in a robust metadata framework is a strategic imperative for organizations managing large volumes of unstructured data. By standardizing metadata practices, leveraging automation tools, and maintaining high-quality metadata, organizations can enhance their data governance and unlock the full potential of their data assets.
As data grows in complexity and volume, the strategic implementation of a metadata framework becomes increasingly essential. It ensures that data handling practices are efficient and aligned with high standards of data quality, interoperability, and security, supporting the development of advanced AI systems and enabling organizations to harness the power of data for transformative outcomes.
By following these essential practices, enterprises can build a robust metadata framework that stands the test of time, fostering a data-centric culture and paving the way for future technological advancements.