Improving RAG Accuracy with Intelligent Metadata Solutions
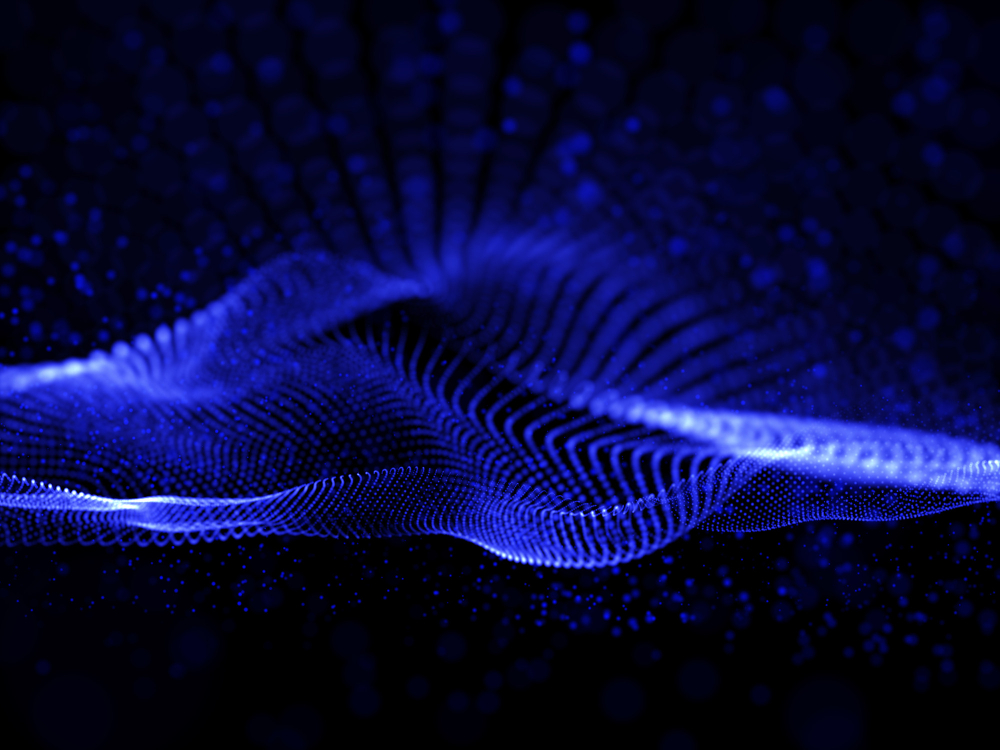
Rapid Annotation and Grounding (RAG) models have been increasingly adopted by enterprises to manage large volumes of unstructured data. Enhancing RAG accuracy through intelligent metadata solutions addresses challenges associated with this type of data. Here, we delve into how metadata contributes to improved precision and efficiency in RAG operations.
Significance of Metadata in RAG
Metadata, encompassing descriptive, structural, and administrative information about data objects, can significantly enhance the performance of RAG models by providing additional context and structure.
Contextual Enrichment
Metadata supplies detailed contextual information that aids in disambiguating data points. For instance, in healthcare applications, metadata such as patient age, medical history, and diagnostic context can improve the accuracy of RAG models in grouping radiographic images . Additionally, metadata can enhance feature learning by adding dimensions over which the model can operate.
Hierarchical Structuring
Implementing hierarchical metadata enables a structured representation of data categories, facilitating more accurate annotations and groupings. By organizing metadata into taxonomies and ontologies, RAG models can effectively learn the relationships between different data classes, enhancing model generalization and precision .
Detailed Case Study: Metadata in Financial Document Processing
Consider a financial services firm aiming to enhance the accuracy of RAG models for processing various financial documents such as transaction reports, invoices, and balance sheets.
Metadata Design
The metadata system was meticulously designed to capture multiple attributes of financial documents, including document type, date of issuance, involved parties, transaction type, and amounts. This design was based on established financial reporting standards to ensure comprehensiveness and relevance.
Implementation
- Annotation Tooling: Tools such as Deasie's automated labeling workflow were deployed to facilitate the annotation process. These tools supported the inclusion of metadata at multiple stages, helping annotators maintain consistency and accuracy .
- Model Adjustment: The RAG model was adapted to handle metadata-enhanced inputs. This involved integrating a hierarchical loss function that penalized misclassifications differently based on metadata attributes .
Results and Analysis
After implementing these changes, the financial services firm observed an 8.2% improvement in RAG accuracy and a 12% reduction in convergence time . Hierarchical metadata guided the model through structured learning processes, enabling better handling of inter-class variability and reducing error propagation .
Technical Considerations for Implementing Metadata Solutions
Several technical considerations must be addressed for the optimal implementation of metadata solutions:
Metadata Consistency and Standardization
Consistency and standardization across metadata attributes are crucial. This can be achieved by basing metadata schemas on industry-specific standards. Inconsistent metadata can confuse models and degrade performance.
Efficient Storage and Retrieval
The storage infrastructure must support rapid retrieval of metadata during model training and inference. Databases optimized for handling hierarchical and relational data structures, such as graph databases, are effective in this regard.
Scalability
The metadata system should be scalable to accommodate growing data volumes and evolving structures. Cloud-based solutions offer the necessary scalability and flexibility, along with robust tools for managing large-scale metadata repositories.
Automation in Metadata Extraction
Automating metadata extraction can streamline the integration process, enabling the rapid annotation of large datasets. Machine learning algorithms, such as Natural Language Processing (NLP) techniques, can be employed to automate the extraction of metadata attributes from unstructured data sources .
Reflecting on the Strategic Importance of Metadata in RAG
Leveraging intelligent metadata solutions is crucial for enterprises seeking to improve the accuracy of RAG models. Metadata offers a rich source of contextual and structural information that can guide models through efficient and precise learning processes. As the volume and complexity of unstructured data continue to grow, the adoption of advanced metadata solutions will be essential for maintaining high levels of accuracy and efficiency in RAG operations.
By investing in robust metadata systems and integrating them seamlessly into the RAG pipeline, organizations can achieve significant improvements in model performance, laying a strong foundation for the development of advanced AI-driven solutions .